Live
- U.R. Pradeep, Rahul Mamkoothathil take oaths as Kerala legislators
- Woman Sentenced to Prison for Killing, Attempting to Eat Cat Amid False Haitian Rumor
- Vehicle movement restored on Cuddalore-Puducherry-Chennai Highway after two days
- Celebrate National Cookie Day 2024: History, Significance, Theme, Messages, Quotes & More
- Massive fires ravage commercial buildings in Ranchi and Giridih, losses run into crores
- Priority is to fulfil promises, take Maha to top level in all sectors: Devendra Fadnavis
- India’s Favorite Beer Just Got Better; Find Out Why This Brand Reigns Supreme
- International Bank Day 2024: Empowering sustainable growth through finance
- Bull rally takes valuation of BSE-listed companies above Rs 450 lakh crore
- Manipur: Inter-district public transport resumes in six districts after 19 months
Just In
Siddhant Benadikar: Innovating the Future of Computing
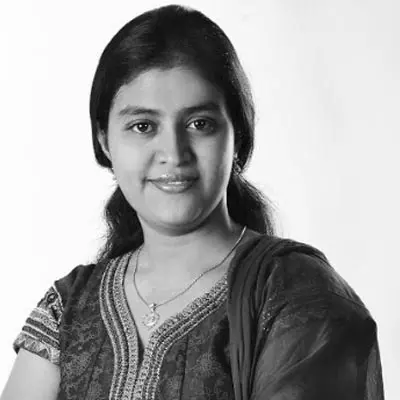
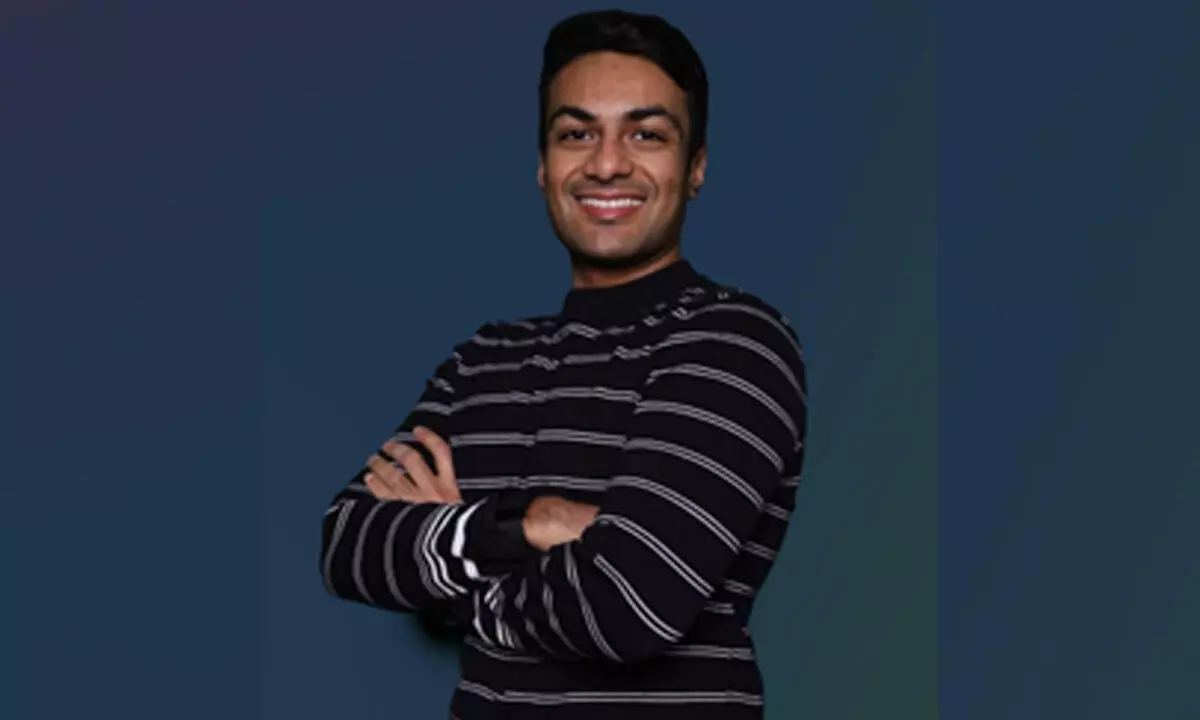
Siddhant Benadikar is a visionary software engineer whose work in distributed systems and graph computing has led to groundbreaking innovations. With experience at Meta and QuickBase, he is shaping the future of technology through his expertise and leadership. He talks about his journey and how his innovative work in distributed systems and graph algorithms is set to shape the future of computing
Siddhant Benadikar is a forward-thinking software engineer whose work spans distributed systems, graph learning, and graph computing systems. With a robust educational background from Northeastern University and the University of Mumbai, coupled with extensive experience at tech giants like Meta and QuickBase, Inc., Siddhant's journey is nothing short of inspiring. He holds patents for innovative solutions in offline structured data entry and navigation on mobile devices, making significant strides in the tech world.
Q1: Your journey in tech is impressive, especially your work in graph algorithms and computer systems. Can you share what initially drew you to these areas?
A: My interest in graph algorithms and distributed computing systems began during my undergraduate studies at the University of Mumbai. The way graph theory could be applied to solve real-world problems intrigued me. The potential to enhance data analysis and decision-making processes through graph algorithms has always driven my passion. At Northeastern University, I delved deeper into distributed systems for parallel data processing such as Spark, which became the subject of my thesis. Later at Meta, I had the unique opportunity to merge my two areas of fascination: distributed compute systems and graph theory. This led me to join the team that specializes in these fields. The potential to enhance data analysis and decision-making processes through graph algorithms has always driven my passion.
Q2: At Meta, you spearheaded the implementation of new graph algorithms for recommendation systems. What was the most challenging aspect of this project?
A: At Meta, the size of the graphs are in the order of Billions of nodes and trillions of edges. The most challenging aspect was optimizing the algorithms to handle the massive scale of data we were dealing with. We needed to ensure that the system could process and analyze vast amounts of information quickly and efficiently. This involved minimizing memory footprint, implementing data spilling to disk, and ensuring the algorithms could scale vertically. Balancing performance with accuracy was a constant challenge.
Q3: OneGraph, the offline graph compute system you prototyped, is a significant achievement.
How do you envision its impact on future advancements in graph computing?
A: OneGraph has the potential to revolutionize how we handle large-scale graph computations. It enables execution of complex graph algorithms on Spark, capitalizing on Spark's scalability and reliability. Additionally, it accelerates computation, which is crucial given the iterative nature of graph algorithms... This can lead to advancements in fields like social network analysis, recommendation systems, and even biological network analysis. The groundwork we’ve laid with OneGraph can pave the way for more robust and scalable graph computing solutions.
Q4: You amplified Digraph's scale by 5x, enabling it to execute complex algorithms for massive graphs. Can you elaborate on the techniques you used to achieve this?
A: The key techniques involved optimizing the memory usage and implementing efficient data spilling mechanisms. By reducing the memory footprint, we could process larger datasets. Additionally, using disk-based storage for intermediate data allowed us to handle graphs with trillions of edges. These optimizations, combined with parallel processing techniques, enabled us to scale Digraph significantly.
Q5: Mentoring is a crucial aspect of your role. What strategies do you employ to ensure your mentees transition successfully into full-time roles?
A: Mentorship is about providing both technical guidance and personal development. I focus on regular code reviews, weekly knowledge-sharing sessions, and encouraging a growth mindset. It’s important to create a supportive environment where mentees feel comfortable asking questions and taking on challenges. Providing constructive feedback and celebrating their achievements also play a significant role in their successful transition.
Q6: At QuickBase, you developed scalable and resilient ingest pipelines. What were the key considerations in designing these pipelines?
A: Scalability and resilience were the primary considerations. We needed the pipelines to handle varying data loads without compromising performance. Utilizing AWS managed services like API Gateway, AmazonMQ, and FluentD helped us build a robust infrastructure. Cost efficiency was another critical factor, which we achieved by opting for solutions like S3, Kinesis streams, and Firehose over more expensive alternatives like Kafka.
Q7: Your work on the data streaming solution at QuickBase proved more cost-effective than Kafka. What lessons did you learn from this project?
A: The biggest lesson was the importance of evaluating all available options before committing to a solution. By thoroughly understanding the requirements and constraints, we were able to choose a more cost-effective and efficient solution. Flexibility and adaptability are crucial when designing systems that need to evolve with changing needs and technologies.
Q8: Can you tell us more about the patents you hold and the innovation behind them?
A: The patents are for offline structured data entry and navigation on mobile devices. The innovation lies in providing users with the ability to access and enter data even when they are offline, ensuring seamless continuity. This was achieved by developing efficient data synchronization mechanisms and optimizing the mobile app’s architecture to handle offline operations effectively. The result is a significant enhancement in user experience and data accessibility.
Q9: How do you stay updated with the latest advancements in your field, and how do you incorporate them into your work?
A: Staying updated requires a continuous learning mindset. I regularly attend conferences, participate in webinars, and follow leading tech journals and blogs. Additionally, I contribute to the community by judging awards and hackathons, which exposes me to new research and development areas. Networking with peers and participating in tech communities also helps. Incorporating new advancements into my work involves experimenting with new technologies and approaches, assessing their feasibility, and integrating them into existing systems to improve efficiency and performance.
Q10: Looking ahead, what are your aspirations for the future, both personally and professionally?
A: Professionally, I am committed to advancing the frontiers of distributed systems and graph computing. With the rising prominence of graph learning and GNNs in both academia and industry, I am eager to explore these areas more deeply. My goal is to lead projects that significantly influence how businesses and individuals leverage technology, creating tangible impacts.
On a personal level, I strive to balance my career with contributions to the community, particularly through mentorship programs and technology education initiatives. My aim is to inspire and empower the next generation of engineers, fostering a legacy of innovation and knowledge sharing.
Siddhant Benadikar's career exemplifies the relentless pursuit of technological excellence and innovation. His contributions to the development of advanced graph computing systems and his ability to lead and mentor teams have left a significant mark on the industry. With a clear vision for the future and a passion for continuous learning, Siddhant is poised to drive further breakthroughs in the tech world. His journey from academia to leading impactful projects at major tech companies serves as an inspiration to aspiring engineers and highlights the transformative power of dedication and ingenuity. As he continues to push the boundaries of what's possible, Siddhant’s work promises to shape the future of technology and inspire the next generation of tech leaders.

© 2024 Hyderabad Media House Limited/The Hans India. All rights reserved. Powered by hocalwire.com